Mathematical Modeling of Chemical Exposures
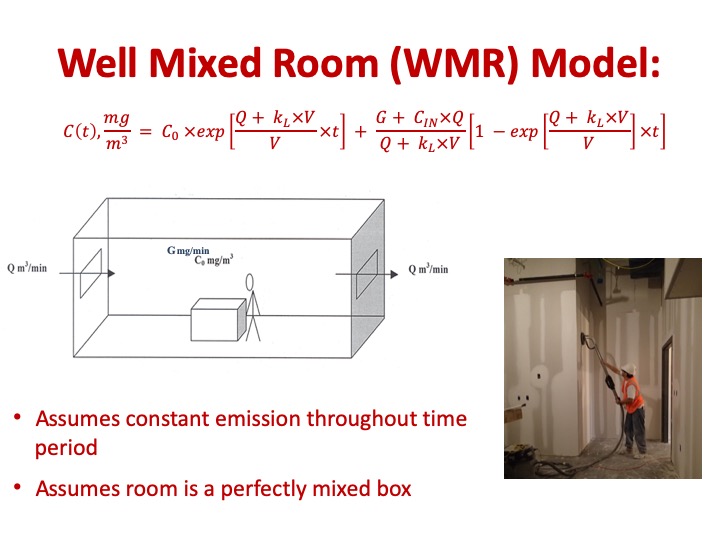
Deterministic physical models are becoming increasingly popular in exposure assessment scenarios to model underlying physical processes in theoretically controlled settings. Experimental data do not arise in theoretically perfect settings and are likely contaminated with measurement error and other external disturbances. Hence, deterministic simulations from the physical model using an arbitrary set of inputs are unlikely to emulate the field data well enough. Thus, there is a critical need for a modeling framework that will relate the field data with the physical models or, more generally, the underlying processes generating the data. Within the healthcare settings, surface contamination by these hazards is well documented but mostly from a drug/patient-safety standpoint. The most common route of exposure is inhalation, although not much is known about the dermal component.
There is a need for predictive exposure models for nanomaterials, as well as models to individually track particles through the environment while keeping track of the particle shape, surface area, and other surface characteristics as the nanoparticles are transformed or become reactive.
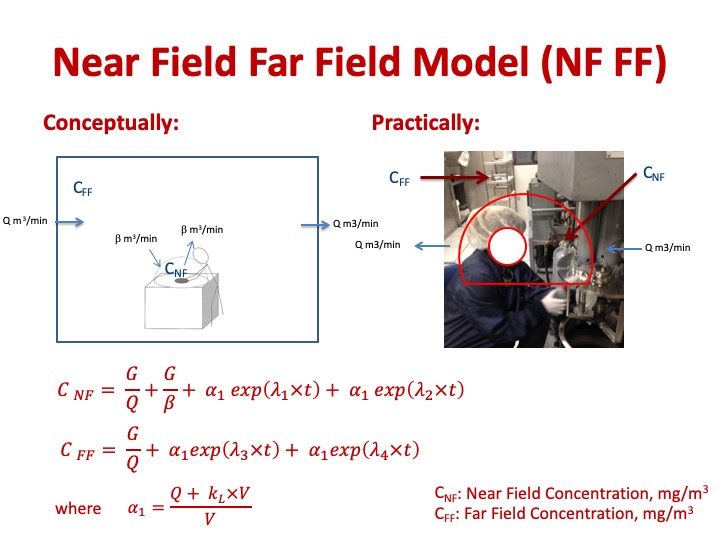
The goal of this research is to develop a suite of mathematical models to estimate worker exposure to nanomaterials as active pharmaceutical ingredients (APIs) or high-potent compound (HPC) in pharmaceutical manufacturing facilities or healthcare facilities that use these drugs and characterize the potential for workers to become exposed and potential health risks associated with this exposure. Further, we propose to model the impact of implementing workplace controls on worker exposure to APIs or HPCs. This will be accomplished through the following aims:z
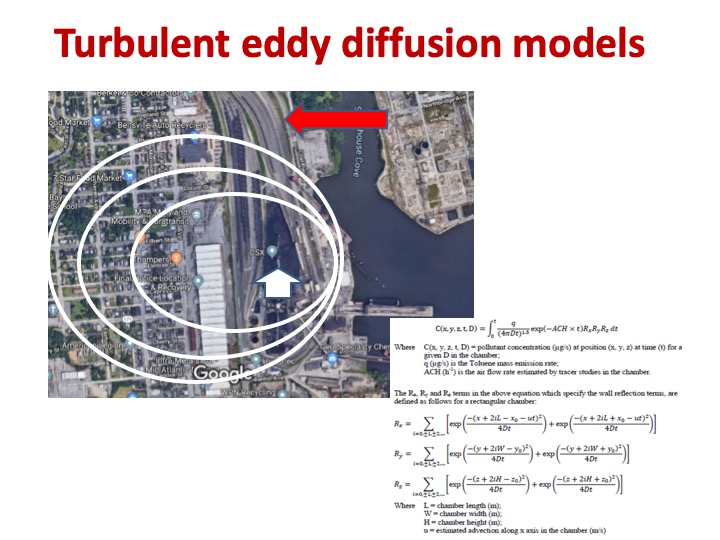
AIM 1: To describe and evaluate a mathematical modeling framework for occupational exposure to nanomaterials and estimate the potential for workplace exposure. We will use (1) well-established inhalation models of exposure (e.g. the well mixed room, near-field/far-field, and eddy diffusion models), (2) dermal models of exposure, (3) detailed nanoparticle dynamics using Markov chain techniques, and (4) Monte Carlo techniques. The model will account for significant determinants of exposure, allowing us to identify effective interventions and quantify the potential reduction in exposure. Furthermore, quantitatively estimating the potential for workplace exposure to nanomaterials will enable us to more effectively conduct occupational exposure assessments.
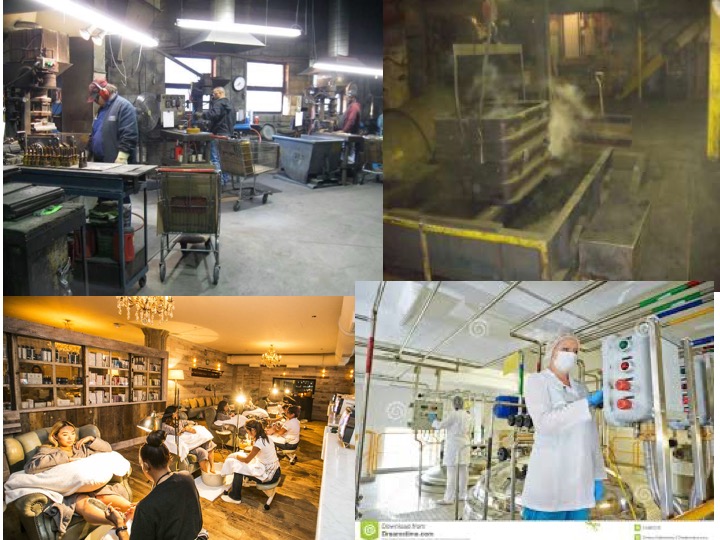
AIM 2: To quantitatively evaluate the predictive exposure model for occupational exposure to nanomaterials in highly-controlled and in actual occupational settings. The effect of donning/doffing personal protective equipment, specific work tasks involving large quantities of nanomaterials, and lab hygiene will be modeled using Markov chains. These tasks will be evaluated in (1) a highly-controlled exposure chamber and (2) in occupational settings where nanomaterials are used (e.g. pharmaceuticals). Results from these exposure assessments will enable us to determine the accuracy of our predictive model and will allow us to develop recommendations to reduce worker exposure to nanomaterials.
AIM 3: To apply the predictive exposure model to nano-scale, high-potent pharmaceutical compounds and to evaluate the health outcome risks associated with occupational exposure to these pharmaceuticals. The model will incorporate occupational exposure estimates into the health risk assessment framework, enabling us to characterize the health risks faced by workers in this industry.
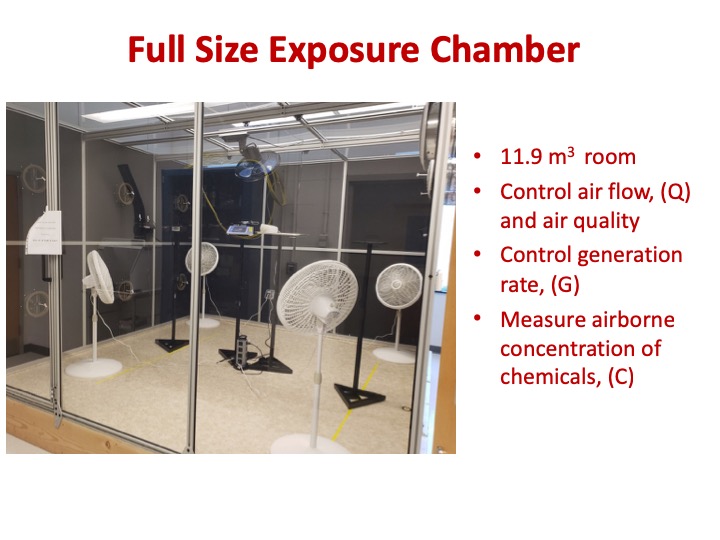