Research and Practice
Research Working Groups
Research in the Department of Biostatistics is organized into the following Working Groups comprised of faculty, postdoctoral fellows, and students. Groups meet regularly in a variety of intellectual meeting formats including research-in-progress sessions, journal club, topical seminars and working discussions. These span population modeling methodologies, “big data” methodologies and applications in both statistical genomics and advanced research technologies such as neuroimaging and wearable computing, causal inference, and the department’s major application areas of environmental health and epidemiology and aging. Faculty and students disseminate their work through publications, software, blogs, and other avenues.
Our research is characterized by a commitment to statistical science, its foundations and methods, and the application of statistical science to the solution of public health and biomedical problems. Research that occurs at the interface of quantitative reasoning and important public health and biomedical questions is particularly potent. We are fortunate to have the opportunity to build our research efforts on the foundation of first-rate biomedical discoveries made here at Johns Hopkins.
For additional information, please read more about our research areas and visit the websites of our Working Groups listed below.
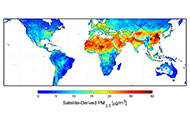
Bayesian Learning & Spatio-temporal modeling (BLAST)
Bayesian and spatio-temporal models provide principled approaches to dealing with complex structures underlying modern large-scale data, yet major methodological and computational challenges remain in their practical deployment. The BLAST group explores ideas and innovations necessary to meet these challenges. Application areas of interest include, but are not limited to, precision medicine, environmental health, disease epidemiology, genetics, healthcare analytics, etc.
Learn more about BLAST. For further information and/or to sign up for our mailing list, contact group leaders.
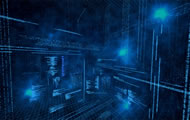
Causal Inference
This group is comprised of a multi-disciplinary group of students, postdoctoral fellows, and faculty from Johns Hopkins University, who are interested in the application and development of statistical methods for drawing causal inferences about intervention effects from partially-controlled studies, or from randomized controlled trials with complications such as non-compliance or missing data. We host open group meetings approximately once per month, with speakers.
Learn more about Causal Inference.
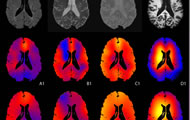
Statistical Methods & Applications for Research in Technology (SMART)
The SMART group works on statistically principled methods for new technologies with special emphasis on brain imaging (e.g. fMRI, high resolution MRI, CT), wearable computing (e.g. hip accelerometers, heart monitors), and Biosignals (e.g. EEG, EKG, ECoG). The underlying principle is to develop methods that are automated, fast, scalable, and robust (AFSR.) Our analytic approaches are sometimes focused only on one subject, but typically we are investigating large populations observed at one or multiple time points.
Learn more about SMART.
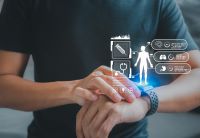
Wearable and Implantable Technology (WIT)
The Wearable & Implantable Technology (WIT) research group is focused on adapting existing methods when possible and developing new methods when necessary to analyze data generated by wearable sensors used in health studies. Such sensors produce very large, high-resolution, and high-heterogeneity data that capture a variety of health-related characteristics including objectively measured physical activity, blood glucose, and blood pressure. Such data are increasingly integrated with ecological momentary assessment (fast recall-based information obtained from digital apps) and are changing both the scientific and biostatistics research environments.
Contact Ciprian Crainiceanu for more information.
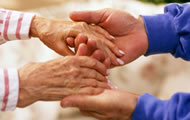
Epidemiology & Biostatistics of Aging (EBA)
The bi-weekly EBA Research-in-Progress meetings involve trainees, faculty members, and others in the Johns Hopkins Schools of Public Health, Medicine and Nursing interested in the power of quantitative thinking to improve health outcomes in aging. The sessions serve to develop trainees’ critical thinking and oral communication skills, as well as knowledge of issues integral to aging research and career development. Each trainee presents an annual formal seminar during their training period.
Learn more about the Epidemiology and Biostatistics of Aging Training Program.
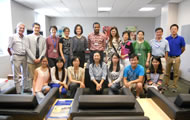
Survival, Longitudinal & Multivariate Data (SLAM)
This working group is a forum for discussion of state of the art research in Survival, Longitudinal, And Multivariate data (SLAM). Established in 1997 under a different name, SLAM was renamed in 2005 to emphasize research on survival, longitudinal, and multivariate data and statistical inference. SLAM activities usually take the following formats: seminar presentations; short courses offered by faculty members; student project presentations.
Learn more about SLAM.
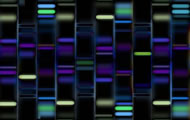
Genomics Data Science Working Group
The Genomic Data Science Working Group welcomes all students, postdoctoral fellows, and faculty who are interested in learning more about areas of research in Genomic Data Science. Our working group is designed to discuss emerging research topics related to genomic data science and statistical genomics. It is a mix of presentations from students and postdocs along with external speakers. We aim to provide a welcoming, intellectually stimulating, and inclusive experience for everyone. Our meeting schedule is posted in the #working_group_genomics channel in the JHU Biostat Slack workspace.
Learn more about the Genomics Data Science Working Group.
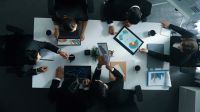
Statistical Practice & Research Collaboration (SPARC)
The Statistical Practice and Research Collaboration (SPARC) working group serves as a learning and exchange hub in this area for our students, postdoctoral fellows, and faculty, as well as statisticians from other Johns Hopkins and Bloomberg School of Public Health departments and centers. SPARC aims to harness the extensive knowledge and experience of our faculty and collaborators to improve our statistical practices and research collaborations, and to prepare our students for a successful career in academia, government, or industry.
Contact Jiangxia Wang for more information.

Statistical Genetics
The mission of the Statistical Genetics Working Group (SGWG) is to build a forum for discussions and collaborations among Johns Hopkins researchers who are interested in cutting edge developments in methodological and theoretical statistics, and related computations, motivated from modern large-scale genetic studies. The group currently meets every alternative week and invites a mix of internal and external speakers for seminar presentations. SGWG also holds join meetings with other working groups in the Biostatistics Department on topics of mutual interest.
Contact Nilanjan Chatterjee for more information.
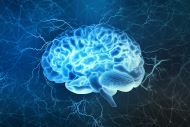
Functional Neuroimaging
The functional neuroimaging group is a multi-disciplinary group of students and faculty from Johns Hopkins University that work on developing new methods for using neuroimaging technology to measure brain function.
Contact Martin A. Lindquist for more information.
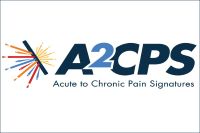
Pain Data Science
Understanding the mechanisms underlying the transition to chronic pain is key to mitigating the dual epidemics of chronic pain and opioid use in the U.S. As part of the NIH funded Acute to Chronic Pain Signatures (A2CPS) Consortium, we have established a Data Integration and Resource Center (DIRC) at Johns Hopkins University and collaborating institutions that works to integrate imaging, omics, behavioral, and clinical measures to develop biosignatures for the transition to chronic pain.
Learn more about Pain Data Science.