New Method Can Improve Assessing Genetic Risks For Non-White Populations
Scalable AI-based method makes use of genetic studies that include people of different ethnic backgrounds and could one day help address health disparities.
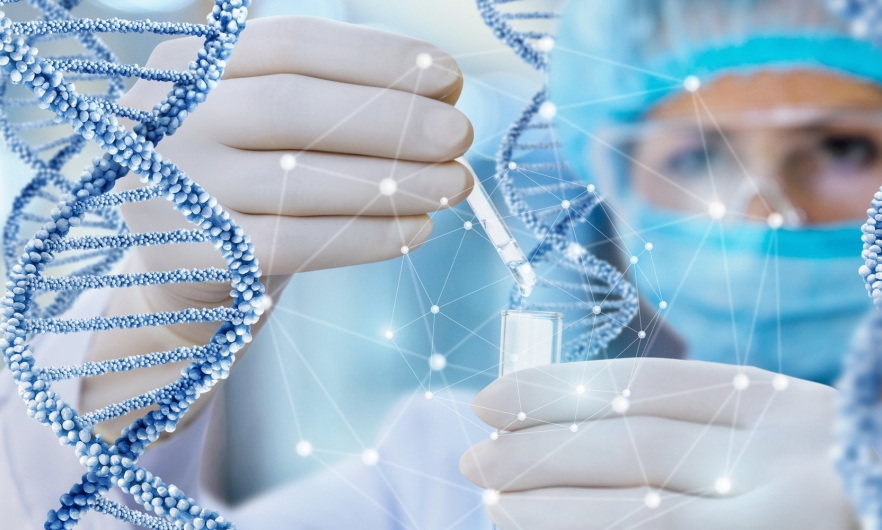
A team led by researchers at Johns Hopkins Bloomberg School of Public Health and the National Cancer Institute has developed a new algorithm for genetic risk-scoring for major diseases across diverse ancestry populations that holds promise for reducing health care disparities.
Genetic risk-scoring algorithms are considered a promising method to identify high-risk groups of individuals who could benefit from preventive interventions for various diseases and conditions, such as cancers and heart diseases. These risk-scoring algorithms are based on large-scale genetic studies that link certain DNA variants to higher or lower disease risks.
The vast majority of subjects in these genetic studies have been people of European ancestry. The resulting risk-scoring algorithms have not always performed well in other populations, due to genetic differences across populations.
The new method, described in a paper that appears online today in Nature Genetics, has been applied to data from genetic studies from 23andMe Inc. and other sources involving more than 5 million individuals across diverse populations to generate genetic scores for 13 traits, including health conditions like coronary artery diseases and depression, in five different ancestry categories: European, African, Latino, East Asian, and South Asian. The researchers also tested the new method in large-scale simulation studies.
“We showed that our method can help close the risk-scoring performance gap for non-European-ancestry populations,” says study senior author Nilanjan Chatterjee, PhD, Bloomberg Distinguished Professor in the Bloomberg School’s Department of Biostatistics. “At the same time, we also concluded that we can’t fully close the gap with new methods alone—we also need larger datasets on these populations.”
Many risk-scoring models derived from genetic studies in non-European-ancestry populations often fall short because those studies typically are relatively small in scale. This results in a performance gap in risk-scoring between European-ancestry and other-ancestry populations, which may contribute to health care disparities.
The new method—which the researchers call CT-SLEB—used a combination of AI techniques including machine learning and Bayesian statistical modeling. In addition to the 23andMe database, the researchers “trained” CT-SLEB on data from the Global Lipids Genetics Consortium, the National Institutes of Health’s All of Us research program, and UK Biobank.
The research team’s benchmarking analyses showed that these new ancestry-specific risk-scoring models for the non-European populations generally outperformed standard polygenic risk score models that are based on mostly European-ancestry datasets, or are based on smaller non-European-ancestry datasets.
The researchers also compared CT-SLEB to a number of alternative methods. They found the proposed method is particularly helpful to improve genetic risk scores in African ancestry populations where scoring accuracy is generally the lowest. The team also found that CT-SLEB is computationally much faster compared to its closest competitors, and thus could be amenable to analyzing much larger numbers of DNA variants and more populations.
The team is now working with more advanced methods that are even better performing but are still computationally fast, Chatterjee says.
He also emphasizes that, as the team’s calculations in the study showed, having polygenic risk score models that work equally well in non-European-ancestry and European-ancestry populations will require more genome-wide association studies in non-European-ancestry populations.
“A lot of people think machine-learning and AI can do magic but without large, well-designed studies, algorithms will not be as useful,” Chatterjee says.
The paper’s lead author is Haoyu Zhang, PhD, who was a doctoral student at the Bloomberg School at the time the study began and is currently an investigator at the National Cancer Institute. Researchers from 23andMe contributed to development of the new method and the analysis of the data. The CT-SLEB code is publicly available via GitHub. The code availability section in the paper includes a link to GitHub which includes the CT-SLEB code.
“A new method for multiancestry polygenic prediction improves performance across diverse populations” was co-authored by Haoyu Zhang, Jianan Zhan, Jin Jin, Jingning Zhang, Wenxuan Lu, Ruzhang Zhao, Thomas Ahearn, Zhi Yu, Jared O’Connell, Yunxuan Jiang, Tony Chen, Dayne Okuhara, 23andMe Research Team, Montserrat Garcia-Closas, Xihong Lin, Bertram Koelsch, and Nilanjan Chatterjee.
Funding was provided by the National Institutes of Health (K99 CA256513-01, R00 HG012223, 5T32HL007604-37, R35-CA197449, U19-CA203654, R01-HL163560, U01-HG009088, U01-HG012064, R01 HG010480-01 and U01HG011724).
# # #
Media contacts: Kris Henry khenry39@jhu.edu and Barbara Benham bbenham1@jhu.edu