How Health Care Algorithms and AI Can Help and Harm
Algorithms can amplify health inequities—or help find biases in medical data.
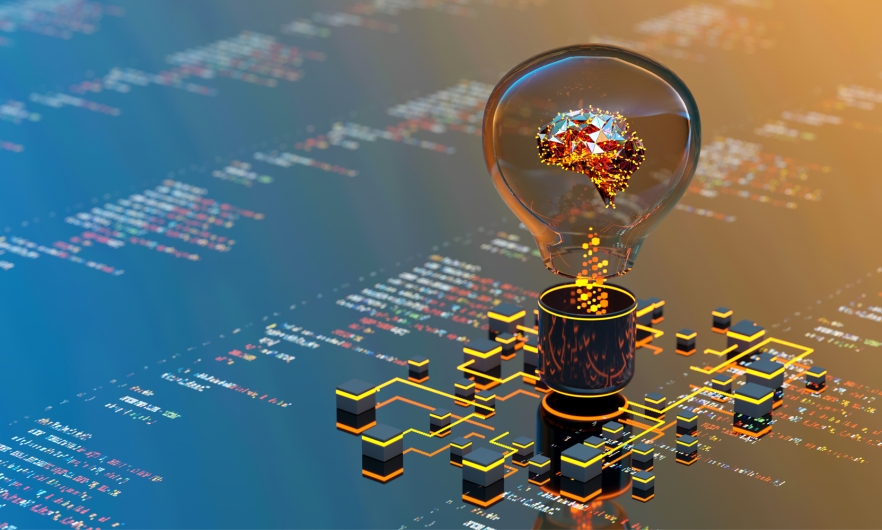
When we think of potentially dangerous, unseen challenges to public health, pathogens and toxins often come to mind. But algorithms—formulas that do everything from suggesting Netflix shows to presenting Google results—are increasingly used in health care settings, and could be amplifying harmful biases and exacerbating health disparities.
In this Q&A, adapted from the April 17 episode of Public Health On Call, Joshua Sharfstein, MD, speaks with Kadija Ferryman, PhD, assistant professor of Health Policy and Management and core faculty at the Berman Institute of Bioethics, about what algorithms are and the double-edged sword of their use in medicine.
What's an algorithm?
Think about it as a recipe—a set of instructions. If you're going to make dinner, you look at a list of ingredients and the steps of what you need to do. That in a way is akin to what algorithms are. There are sets of ingredients, which we think of as data that we need to make the algorithm run. And then there are a set of instructions that tell that computer what to do with the data.
How do algorithms affect our lives?
Algorithms are everywhere. Think of streaming services like Netflix, for example. We can infer from what we see that Netflix's algorithms work by taking the information of what we watch, and then ranking those shows, and showing us things we might like, based on what we've watched before.
There are algorithms in online shopping. If you search for something on Amazon, algorithms in the background sort the things you might be interested in and even after you buy something, show you other things you might want. Even Google search operates using algorithms that try to sort information and present it to the user [in a way that] will be relevant.
Could you give some examples of algorithms that affect our health?
Just like with the Netflix example of recommending things that you might like, in health care settings, there are algorithms that recommend to physicians, nurses, and other kinds of clinicians, the treatments that they should offer and tests that they should run based on the patient’s information.
Algorithms are also used in health care for administrative functions, like scheduling appointments. Other algorithms are used to look at drugs to see if there are new ways that ingredients in a particular drug could be used for another set of conditions.
How are these health care algorithms making their suggestions?
I think that's where some of the concern around algorithms in the health care space comes up. Algorithms in general are often described as being “black box,” meaning that we users—the people on whom the algorithms are used—can't know which pieces of information are being used to make recommendations, how different information is weighted, and which information is included or excluded.
There is an opacity to these algorithms, so even though some of them can be very useful in making recommendations for a test that could be done for a patient or treatment modalities that haven't been considered, there is some concern from clinicians about where the recommendation is coming from and whether they can really trust it.
Because we're talking about treatments and screening, if there is less knowledge about these recommendation tools, it's a little bit more concerning in the healthcare space. There are ways that algorithms are being used by insurance companies, for example, to make recommendations about who could or could not receive extra healthcare resources, for who may be getting sicker in the future. How much agency do people who are subject to those decisions really have in knowing how the decisions came about?
You and your colleagues recently wrote about an example of an algorithm that's widely used when patients report having pain.
We wrote about the NARX score—a risk score used to assess an individual's risk for opioid misuse. We raised questions like, what data points are used to develop this risk score? Could some of those data points be unintentionally making that algorithm biased against certain groups? We wrote about wanting to be cautious about a tool that sounds, on the surface, very necessary and helpful, but could have biases embedded within.
Many algorithms are proprietary. We don't know the exact formulation of the NARX score. But based on other evidence we've gathered, a data point like criminality, for example, is likely to be included. There's racial bias embedded in data on criminal history because of histories of over-policing in certain communities. Often, because of the black box nature of algorithms, a clinician may not know the reasons behind the NARX score their patient had received, and the patient may not know how the algorithm determined their risk level.
Why is algorithmic bias so concerning?
Even though the algorithms are black box and we may not know why the algorithm is making the decisions it is, patterns of disenfranchisement and of differential treatment are repeating historical patterns of disparity. For example, before the use of something like a NARX score, there was research showing that Black patients are denied certain pain medications more often than white patients. With the introduction of algorithms, we see an amplification of already existing patterns of health inequity.
What do you think can be done to address bias in algorithms?
There's no easy answer, but one main strategy is transparency—trying to open up that black box and get a sense of what data points these algorithms are using. How are they making these decisions? What data points are being weighed? How can we document that there are inequitable impacts in different groups?
We can imagine these tools being used in medical practice and in other parts of health care, and we don't know that algorithms are exhibiting this pattern of disenfranchisement of harm between groups. Once we have identified that there are disproportionate harms, we try to get some transparency on the data that's being used and the way that that data is being processed by those algorithms. There's important research that computer scientists are doing to determine how we identify bias in algorithms, and how to de-bias them.
There's also another line of thinking on this that asks, why are we developing the algorithms that we're developing? Which questions are getting funding for development? We think some of the algorithms that are being developed are the ones physicians and other clinicians think are the most important, but often, it's the algorithms for which developers have the most data they can use.
In theory could well-designed algorithms help to address questions of bias?
Absolutely. There's a great paper by colleagues of mine called “Treating Health Disparities with Artificial Intelligence” that’s based on the idea that artificial intelligence tools are really good at certain things, like detecting patterns in data—such as bias or histories of policing in Black and brown communities.
If we know that algorithms and artificial intelligence tools are really good at spotting patterns in data, then we can actually use algorithms to look at our data to find these different patterns. Where do we see differences between groups in treatment, in testing, in access to health care? We can actually use AI as a hypothesis-generating tool to look at biases in the data and help tell us [more] about some of the inequities that we already know exist in health care.